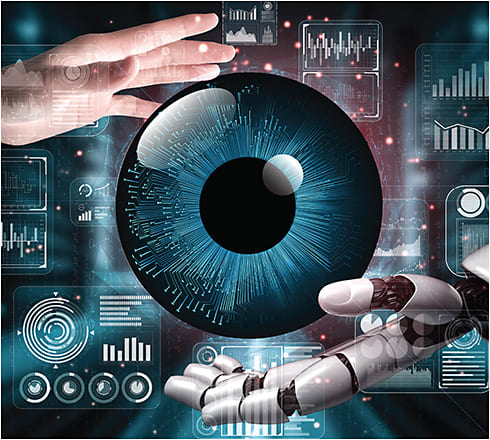
The Sci-Fi film genre is rife with stories of artificial intelligence, or AI, taking over. HAL commandeering the Discovery space station from astronaut Dave Bowman in “2001: A Space Odyssey,” is one such example. “The Matrix,” which reveals a future where humans are obsolete, is another. Arguably, such films have fueled the fear of AI, particularly when it comes to occupations. With regards to the recent availability of AI in eye care, however, both the currently available and in-the-pipeline AI diagnostic and screening technologies appear to aid in clinical decision making (enhancing patient care), provide time savings for doctors and patients, save clinic space, and offer relief for those who have mobility issues.
Additionally, the “national density of ophthalmologists has decreased, while the density of optometrists has increased.”1 It can be argued, then, that ODs are likely to gain referrals from primary-care physicians, among others who employ eye care-related AI.
In this article, we’ll review some of the latest advancements in AI. To be clear, AI in this article is defined as a system that automatically detects and measures features of the eye. (A caveat: AI is a fast-moving field, so please email jennifer.kirby@pentavisionmedia.com with any additions, so the article can be updated online.)
IDENTIFYING AMD
As many as 11 million people in the United States have some form of age-related macular degeneration (AMD), with this number expected to be close to 22 million by 2050.2
We are well aware that early detection is crucial to preventing vision loss, and so are innovators in ophthalmic AI.
Specifically, a fully automated software is available that employs an AI-based tool to assess retinal fundus color images for AMD. Called iPredict AMD, its manufacturer, iHealthScreen, says it has been 88.7% accurate on more than 500 fundus images in identifying “referrable AMD.”3
Additionally, one systematic review and meta-analysis of 19 studies on AI for the detection of AMD in color fundus photographs reveals it is successful, indicating “the application of AI-based automatic tools is beneficial for the diagnosis of AMD.”4
GRADING/MANAGING CATARACT
A total of 17.2% of Americans older than age 40 have a cataract in at least one eye (about 20.5 million people), reports the Centers for Disease Control. By 2028, over 30 million Americans are likely to have cataracts.5
In-the-pipeline AI for this condition includes grading nuclear cataract severity from slit-lamp images, “assisting and improving clinical management of the disease in the context of large-population screening;”6 an algorithm comprised of image-level classification, anatomical, and pathological labels to aid in diagnosis;7 and a platform for managing cataracts collaboratively, which includes an AI-based medical referral pattern for the improvement of efficiency and resource coverage;8 and convolutional neural networks (CNN) to investigate cataracts layer by layer.9 (CNNs learn task performance via repetition and self-correction.)
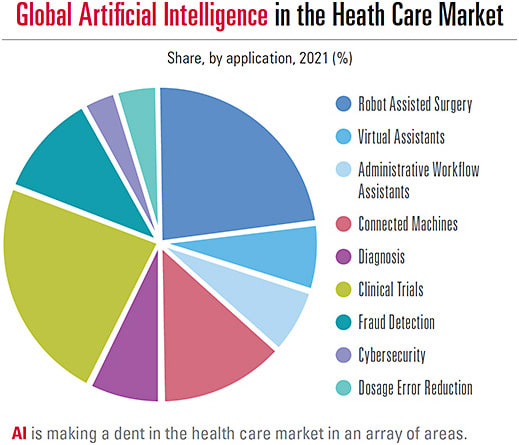
TRIAGING DIABETIC RETINOPATHY
Most patients who have type 1 diabetes, and over half of patients who have type 2 diabetes will develop some form of sight-threatening retinopathy within the first two decades after diagnosis.10 Of note: A total of 34.2 million people of all ages — or 10.5% of the U.S. population — has diabetes.11
Through early detection and treatment, the majority of diabetes-related blindness can be avoided (approximately 90% is preventable).12 That said, it’s no secret that many patients who have diabetes fail to have their eyes checked routinely. (See “Address This Top Challenge in Managing Diabetes,” at bit.ly/DiabetesApts .)
Four AI technologies, which can be employed in non-ophthalmic health care settings, can funnel such patients to eye care providers for further evaluation. They are EyeScreen (Eyenuk, Inc.) iCare ILLUME (Centervue), the IDx-DR (Digital Diagnostics), and iPrevent DR (iHealthScreen).
The EyeScreen assesses retinal images and provides an ICD-10 compliant report to the ECP, who then scrutinizes the images as well. (See www.eyenuk.com/us-en/products/eyescreen-service .)
The iCare ILLUME is comprised of diabetic retinopathy (DR) screening software powered by AI that automatically identifies early signs of disease from images acquired via the iCare DRSplus fundus imaging system, and then classifies the results by severity. (See www.icare-world.com/us/?ref=global .)
The IDx-DR identifies more than mild DR in patients age 22 and older who have diabetes, though no DR diagnosis. It is used with the Topcon NW400 robotic desktop fundus camera. (See www.digitaldiagnostics.com/products/eye-disease/idx-dr .)
The iPrevent DR employs automated software for DR screening, using an AI-based tool on retinal color fundus images. (See https://ihealthscreen.org .)
INTERPRETING DRY EYE DISEASE
The U.S. prevalence of dry eye disease (DED) in the adult population alone is estimated at approximately 5%.13 Additionally, it is one of the most underdiagnosed and undertreated ocular conditions.14
In-the-pipeline AI for DED includes clinical tests, and the interpretation of interferometry, slit-lamp, and meibography images, reports a literature review on the use of AI in DED.14 The study’s authors note, “While initial results are promising, much work is still needed on model development, clinical testing, and standardisation.”
CLASSIFYING AND MONITORING GLAUCOMA
As three million Americans have glaucoma,15 with approximately half unaware they have the condition,15 it’s not surprising that AI technology is also under development to screen for the early identification and management of the silent thief of sight.
For example, Li et al assessed the efficacy of a deep learning system for identifying glaucomatous optic neuropathy, derived from color fundus photographs.16 The result: The AI system had a sensitivity of 95.6% and a specificity of 92.0% in detecting glaucomatous optic neuropathy.16
Additionally, Asaoka et al used a deep learning method to distinguish the VFs of preperimetric open-angle glaucoma patients from the VFs of healthy eyes with “very high accuracy.”17
Also, Niwas, et al. created a fully automated angle-closure glaucoma (ACG) classification algorithm, based on AS-OCT images. Specifically, a complete set of morphological features in ACG, such as exaggerated lens vault, were extracted directly from raw AS-OCT images and delivered into a “classifier.” The classifier’s overall accuracy was 89.2% and 85.12%, using a leave-one-out cross-validation and 10-fold cross-validation method, respectively.18
VA/Hospital Information Systems, community health centers and OD/MD tertiary care centers will, no doubt, greatly benefit from implementing the aforementioned AI technology under development, as it offers time savings for both practitioners and patients alike. For now, optometrists can evaluate having their glaucoma patients wear a silicone soft contact lens called Triggerfish (Sensimed).
Specifically, the device employs a sensor to detect small changes in corneal curvature over a 24-hour period and transmits the data to a portable recorder worn by the patient. The data are then delivered to a computer via Bluetooth for practitioner analysis. Monitoring changes to corneal shape (via special gauges on the lens) correlates with accurate trends in IOP, capturing valuable measurements outside of office hours. (For more on AI in glaucoma, see “Homing in on Testing,” p.43.)
EMPLOYING VIRTUAL REALITY FOR ALL
The use of virtual reality (VR) is no longer reserved for arcade games or theme park rides, as numerous AI headsets are now available to perform vision and ocular health testing, saving clinic space, which can optimize patient flow.
The Heru Platform (Heru, Inc.) provides six vision diagnostic exams to identify progressive, sight-threatening eye conditions, such as AMD, cataracts, and glaucoma. Specifically, the platform offers VFs (suprathreshold and full threshold), contrast sensitivity, color vision (Ishihara and Farnsworth D-15), and dark adaptation. Additionally, the technology ensures the patient’s fixation is always appropriate, resulting in fewer fixation losses. (See www.seeheru.com .)
M&S Technologies’ SmartSystem VR headset enables testing in a fully illuminated room, includes built-in eye tracking for fixation monitoring, and provides 10-2, 24-2, and 30-2 VF and contour stereo testing. (See mstech-eyes.com/assets/uploads/VR_Dealer_Sheet_Final.pdf .)
VisuALL VR devices (Olleyes) comes in three different products (Visual ETS, Visual S, Visual H) offering perimetry, VA, color vision, contrast sensitivity, and low contrast VA testing, an automated comprehensive eye examination suite, and extraocular motility, pupillometry, and VF with eye-tracking testing. (See https://olleyes.com .)
Xenon-VR’s Xenon-1 provides VF testing featuring liquid lens technology that decreases errors, eye-tracking software to improve the accuracy and reliability of test results, and a built-in camera that produces a live video feed, so we can monitor eye position. Commercial availability of the system is expected end of 2022. (See https://xenon-vr.com .)
A FRIEND, NOT A FOE?
While the Sci-Fi film genre has featured AI as the enemy of man, based on what is both currently available and in the pipeline, AI in eye care appears to be more like “The Iron Giant” and “Big Hero 6” vs. HAL in “2001: A Space Odyssey.” OM
REFERENCES
- Feng PW, Ahluwalia A, Feng H, Adelman RA. National Trends in the United States Eye Care Workforce from 1995 to 2017. Am J Ophthalmol. 2020;218:128-135. doi: 10.1016/j.ajo.2020.05.018. Epub 2020 May 21.
- American Academy of Ophthalmology. Artificial Intelligence. AI is poised to revolutionize medicine. An overview of the field, with selected applications in ophthalmology. https://www.aao.org/eyenet/article/artificial-intelligence . (Accessed July 2, 2022).
- BrightFocus Foundation. Age-Related Macular Degeneration: Facts & Figures. https://www.brightfocus.org/macular/article/age-related-macular-facts-figures (Accessed July 2, 2022).
- BusinessWire. iHealthScreen’s Artificial Intelligence (AI) Based Tool Can Accurately Diagnose Age-Related Macular Degeneration (AMD) and Help Prevent Blindness. Prospective Study Presented at the Annual Meeting of the American Academy of Ophthalmology (AAO), November 13-15, 2020. https://www.businesswire.com/news/home/20201130005925/en/iHealthScreen’s-Artificial-Intelligence-AI-Based-Tool-Can-Accurately-Diagnose-Age-Related-Macular-Degeneration-AMD-and-Help-Prevent-Blindness (Accessed July 2, 2022).
- Dong L, Yang Q, Zhang RH, Wei WB. Artificial intelligence for the detection of age-related macular degeneration in color fundus photographs: A systematic review and meta-analysis. EClinicalMedicine. 2021;35:100875. doi: 10.1016/j.eclinm.2021.100875. eCollection 2021 May.
- Centers for Disease Control and Prevention. Common Eye Disorders and Diseases. https://www.cdc.gov/visionhealth/basics/ced/index.html#:~:text=An%20estimated%2020.5%20million%20(17.2,to%2030.1%20million%20by%202020 . (Accessed July 2, 2022).
- Xinting G, Lin S, Wong TY. Automatic Feature Learning to Grade Nuclear Cataracts Based on Deep Learning. IEEE Trans Biomed Eng. 2015;62(11):2693-701. doi: 10.1109/TBME.2015.2444389. Epub 2015 Jun 11.
- L Wangting, Yahan Y, Xhang K, et al. Dense anatomical annotation of slit-lamp images improves the performance of deep learning for the diagnosis of ophthalmic disorders. Nat Biomed Eng. 2020;4(8): 767-777. doi: 10.1038/s41551-020-0577-y. Epub 2020 Jun 22.
- Wu X, Huang Y, Liu Z, et al. Universal artificial intelligence platform for collaborative management of cataracts. Br J Ophthalmol. 2019;103(11):1553-1560. doi: 10.1136/bjophthalmol-2019-314729.
- Xu X, Zhang L, Li J, Guan Y, Zhang L. A Hybrid Global-Local Representation CNN Model for Automatic Cataract Grading. IEEE J Biomed Health Inform. 2020;24(2): 556-567. doi: 10.1109/JBHI.2019.2914690.
- Niwas SI, Lin W, Xiaolong B, et al. Automated anterior segment OCT image analysis for Angle Closure Glaucoma mechanisms classification. Comput Methods Programs Biomed. 2016;130: 65-75. doi: 10.1016/j.cmpb.2016.03.018.
- CDC. National Diabetes Statistics Report. 2020 Estimates of Diabetes and Its Burden in the United States. https://www.cdc.gov/diabetes/pdfs/data/statistics/national-diabetes-statistics-report.pdf . (Accessed June 30, 2022).
- CDC. Fast Facts of Common Eye Disorders. https://www.cdc.gov/visionhealth/basics/ced/fastfacts.htm . (Accessed June 30, 2022)
- Hakim FE, Farooq AV. Dry Eye Disease: An Update in 2022. JAMA. 2022;(5): 478-479. doi: 10.1001/jama.2021.19963.
- Storas AM, Strumke I, Riegler MA, et al. Artificial intelligence in dry eye disease. Ocul Surf. 2022 Jan;23:74-86. doi: 10.1016/j.jtos.2021.11.004. Epub 2021 Nov 27.
- CDC. Don't Let Glaucoma Steal Your Sight! https://www.cdc.gov/visionhealth/resources/features/glaucoma-awareness.html . (Accessed June 30, 2022).
- Li Z, He Y, Keel S, Meng W, Chang RT, He Mingguang. Efficacy of a Deep Learning System for Detecting Glaucomatous Optic Neuropathy Based on Color Fundus Photographs. Ophthalmology. 2016;123(9):1974-80. doi: 10.1016/j.ophtha.2016.05.029. Epub 2016 Jul 7.
- Asaoka R, Murata H, Iwase A, Araie M. Detecting Preperimetric Glaucoma with Standard Automated Perimetry Using a Deep Learning Classifier. DOI: 10.1016/j.ophtha.2016.05.029
- Niwas SI, Lin W, Bai X, et al. Automated anterior segment OCT image analysis for Angle Closure Glaucoma mechanisms classification. Comput Methods Programs Biomed. 2016;130:65-75. doi: 10.1016/j.cmpb.2016.03.018. Epub 2016 Mar 21.