Real-world evidence (RWE) is a type of clinical evidence that can help improve the data collected from clinical trials. RWE, especially when assisted by artificial intelligence (AI), can serve as a complementary source of evidence for randomized clinical trials (RCTs) and can help capture the complexity of clinical practice. The traditional understanding of RWE, such as observational studies, patient registries, and electronic health records (EHRs), can provide insights into the effectiveness of myopia management strategies in diverse and unselected patient populations; however, they usually suffer from limitations, such as risk of selection bias and confounding, which sometimes yield unreliable results and interpretations. In contrast, an AI-assisted, self-learning RWE emphasizes a dynamic and data-driven approach. These RWEs make use of the key components, which are discussed below along with their benefits to research and care:
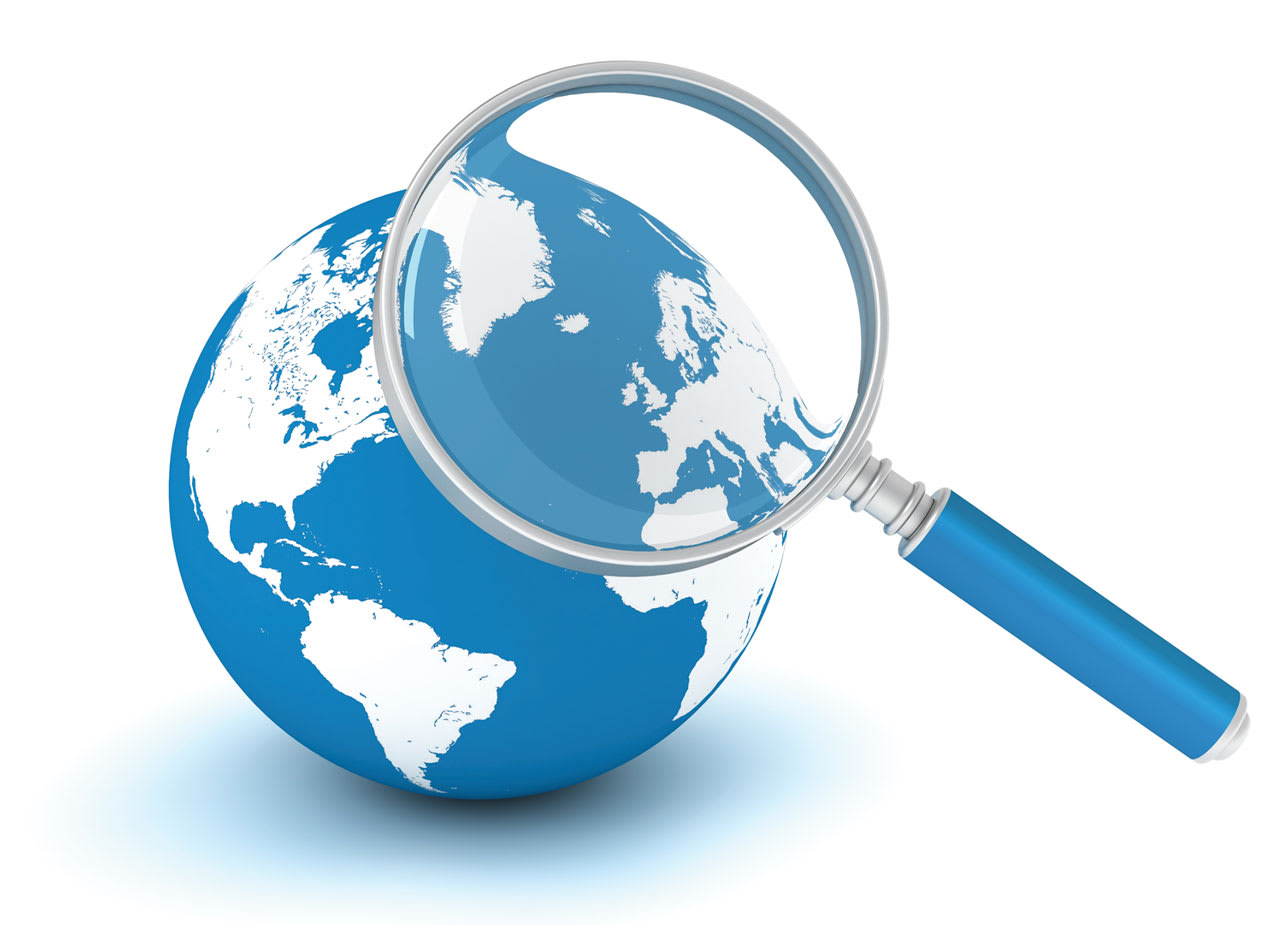
Customized EHR
Customized EHR systems are critical for streamlining the collection of clinical data related to myopia. These systems should be designed to capture relevant information — not only family history, lifestyle factors, and general exam results, but also detailed treatment information, and auxiliary test results such as biometry, pupil profile, and endothelial cells. The ability to gather comprehensive and consistent data lays the foundation for personalized treatment plans and long-term monitoring.
Text/image recognition
Efficient data collection also requires the incorporation of automated text and image recognition technology. Patients often provide valuable information in various formats, including handwritten notes, photographs, and text messages. Utilizing machine learning algorithms for text and image recognition enables health care providers to import and analyze this diverse range of patient-reported data seamlessly. This facilitates real-time data integration, enhancing the quality of clinical decision-making.
Streaming data analysis
Streaming data analysis plays a crucial role in myopia management. Continuous monitoring of a child’s ocular health, visual acuity, and axial elongation allows for early detection of abnormal changes and the need for treatment modification. By analyzing streaming data in real-time, clinicians can predict the most suitable treatment modalities and criteria for adjustment. Algorithms can assess not only well-defined data points, like axial length, corneal curvature, and visual acuity, but also ill-defined data, like retinal images, OCT, and tomography, to adapt treatment plans as needed, ensuring optimal outcomes.
Deep learning models
Deep learning models can further enhance myopia research with the capacity of analyzing large datasets to identify trends, treatment efficacy, and risk factors associated with myopia progression. Compared to traditional statistical approaches, which often rely on predefined models and assumptions about the data distribution, machine learning algorithms can handle complex and high-dimensional data without relying on predefined models or the need to specify the relationships between variables beforehand. Another feature that differentiates machine learning algorithms from traditional statistical methods is its high flexibility in dealing with the nonlinear data or complex interactions between variables, and its adaptiveness to diverse data types and distributions, making them suitable for analyzing various types of eye-related data, including images, genetic data, and clinical measurements. Overall, while traditional statistical approaches have their strengths in hypothesis testing and interpretability, machine learning techniques offer greater flexibility, adaptability, and predictive power, especially when dealing with complex eye-related data. Integrating both approaches can provide complementary insights and enhance the understanding of myopia and its and treatments.
A need for broader evidence
Incorporating AI-assisted RWE can provide a more nuanced understanding of how interventions perform in diverse populations, leading to more effective and personalized patient care. Future research strategies should aim to bridge the gap between RCT evidence and real-world applicability to optimize outcomes in myopia management. OM